There are few, scalable substitutes for the color and nuance that open-ended responses deliver. They help present a more complete picture of the consumer, answering the “why” without the bias of a priori options. In some cases, they’re critical to the research mission itself, as in unaided awareness, recall studies, or analysis of reviews and comments. So why do so many researchers I speak with view open ends as a bit of an afterthought?
Could it be they see all that nice, quantitative data ruined by slang, swears, memes, and misspellings? Or that wealth of information, depth, and nuance locked behind the tedious, time-consuming (and/or expensive) task of coding? Perhaps they’ve just never been able to generate core insights from open-ended questions?
As a result, many insights organizations simply don’t fully utilize the responses from open-ended questions. They may code just a sample, use them only for survey quality control, or just cherry-pick verbatim quotes for their reports. And even when open ends are fully coded, it’s often treated as an appendix, figuratively stapled to the report on its way to the client or leadership and not fully integrated with the insights.
The biggest risk of these approaches is missing critical insights that may be hidden in the unstructured text data of open ends (or in the combination of closed and open ends). It’s worth asking: if analysis of open-ended questions isn’t fully integrated with insights, why are we even asking? Insights professionals must believe there is value to be gleaned from capturing the unfiltered response of respondents, but what does that value look like?
The two types of information gleaned from open-ended questions
To answer this question, we need to consider the essential information that can be understood from open-ended questions. (Canvs has taken a pass at looking at this in a new eBook: The Essential Guide to Insights with Open-Ended Analysis.) We’ve found in our work with both market research providers and in-house insights teams that the answer varies based on the type of research being conducted. But it tends to boil down to two general types of information: Topics and Emotions.
Topics are discrete pieces of information. This could be a product attribute, the talent featured in a program or ad, or even a gameplay element in a video game (eg. a character, skill, or object). And understanding this would be easy enough if humans all communicated in a similar way. But we humans have invented nearly infinite ways of communicating similar concepts, making it difficult to group concepts automatically.
Emotions of course are an expression of how someone feels. Interestingly, I often hear emotion discussed in terms of sentiment of “positive” or “negative”, but these are really value judgments of a particular emotion in a context. For example, “sad” might be negative when discussing a brand, but perfect when a consumer is describing how a “sad” movie made them feel. Our approach at Canvs has been to leave the sentiment value judgment in the hands of the researcher and instead focus on what’s actually being expressed by the consumer.
Capturing emotional nuance
The last several years have demonstrated the importance of empathy for consumer-facing brands. Missing the emotional value expressed in open ends could make the difference between a good campaign and a great campaign.
Of course, these two types of information aren’t mutually exclusive. A single open-ended response may contain both. For example: “I loved the fries, just the right amount of salt and crispiness” and “The whole experience was gross and the workers are super salty.” These verbatims express both topical information and emotion, but they’re also good examples of why surface-level text analysis often fails.
Imagine these are the responses from the quick-serve restaurant (QSR) consumer feedback survey. In one instance, the customer loves the amount of salt on the fries, and in the other, they dislike the amount of salt on the workers. These wonderfully detailed and varied insights about the experience are not likely to be captured with fidelity by the closed-ended questions of your NPS survey.
Integrating open ends into your research
Assuming you agree that open ends represent a meaningful source of insight (or could if they were fully analyzed), consider a thought experiment: how would you approach open-ended questions if there were no limits on your ability to fully analyze them and fully incorporate that analysis into your research?
Perhaps a version of this hypothetical is what’s driving text analytics to be recognized as the top “emerging” method in GreenBook’s 2022 Insights Practice GRIT Report. This is a hopeful sign from my perspective because it not only suggests that insights professionals are increasingly appreciating the value of unstructured text data as a source of insights, but also an increasing focus on developing consumer empathy as a competitive advantage.
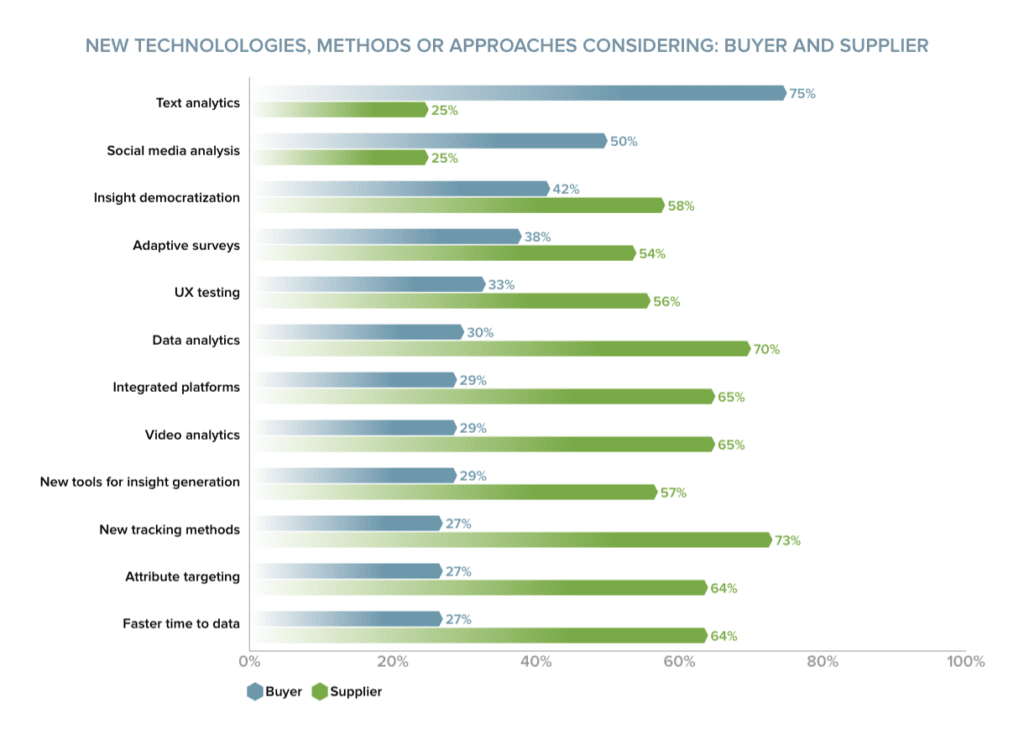
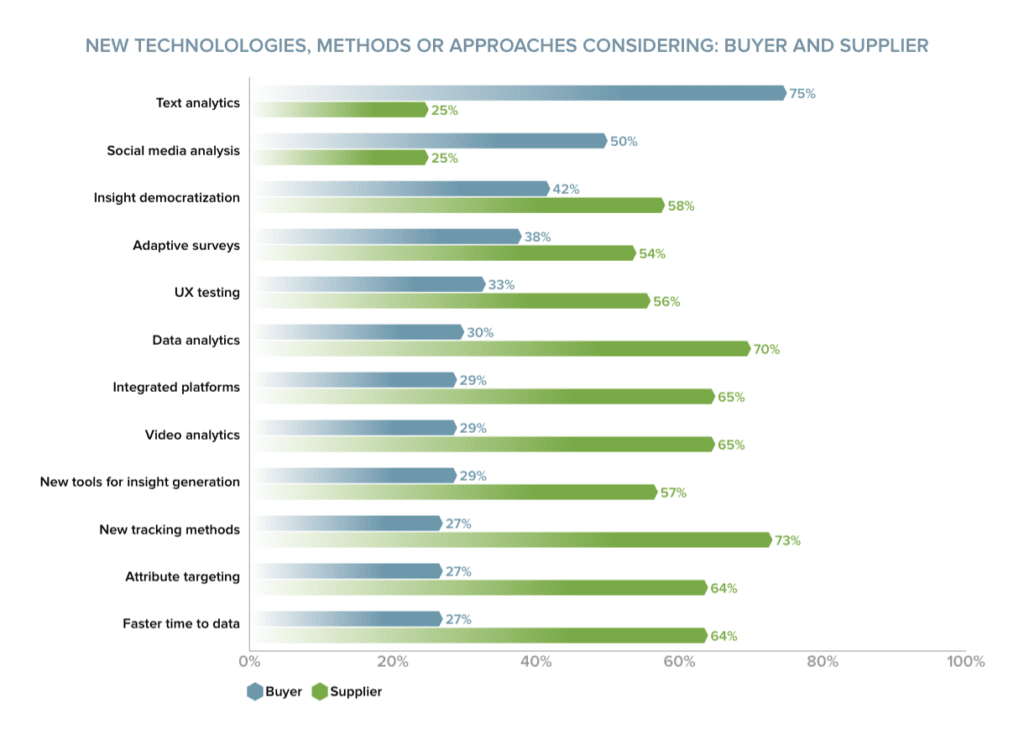
When it comes to text analytics, research technology like Canvs AI is giving researchers the power to bring quantitative scale and statistical significance to the challenge of open-ended responses. This has the potential to not only enhance analytical confidence, but also to expand the potential for open-ended questions in research. The potential for analysis of open-ended text to reach its potential to deliver core insights.
If you’re interested in learning more about analysis of open-ended questions, please download the latest eBook from Canvs: The Essential Guide to Insights with Open-Ended Analysis.